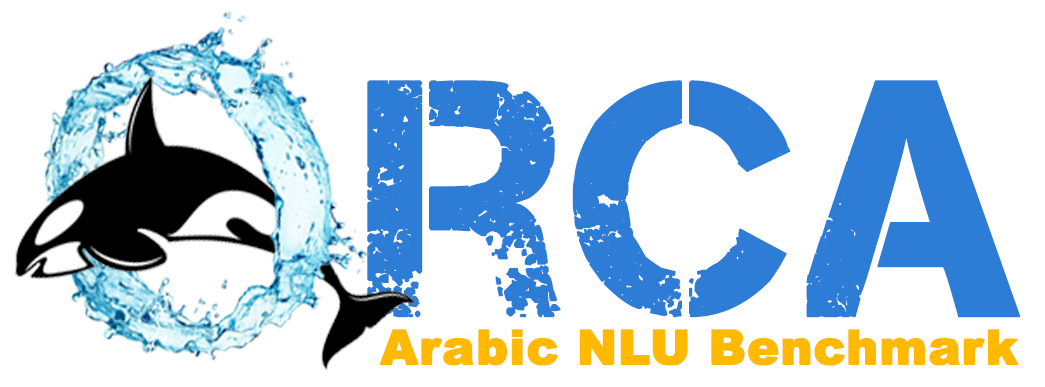
Introduction
Due to their crucial role in all NLP, several benchmarks have been proposed to evaluate pretrained language models. In spite of these efforts, no public benchmark of diverse nature currently exists for evaluation of Arabic. This makes it challenging to measure progress for both Arabic and multilingual language models. This challenge is compounded by the fact that any benchmark targeting Arabic needs to take into account the fact that Arabic is not a single language but rather a collection of languages and varieties. In this work, we introduce ORCA, a publicly available benchmark for Arabic language understanding evaluation. ORCA is carefully constructed to cover diverse Arabic varieties and a wide range of challenging Arabic understanding tasks exploiting 60 different datasets across seven NLU task clusters. To measure current progress in Arabic NLU, we use ORCA to offer a comprehensive comparison between 18 multilingual and Arabic language models.
Citation
If you use ORCA benchmark for your scientific publication, or if you find the resources in this website useful, please cite our paper as follows.
@inproceedings{elmadany-etal-2023-orca, title = "{ORCA}: A Challenging Benchmark for {A}rabic Language Understanding", author = "Elmadany, AbdelRahim and Nagoudi, ElMoatez Billah and Abdul-Mageed, Muhammad", booktitle = "Findings of the Association for Computational Linguistics: ACL 2023", month = jul, year = "2023", address = "Toronto, Canada", publisher = "Association for Computational Linguistics", url = "https://aclanthology.org/2023.findings-acl.609", pages = "9559--9586", }